04 July 2024
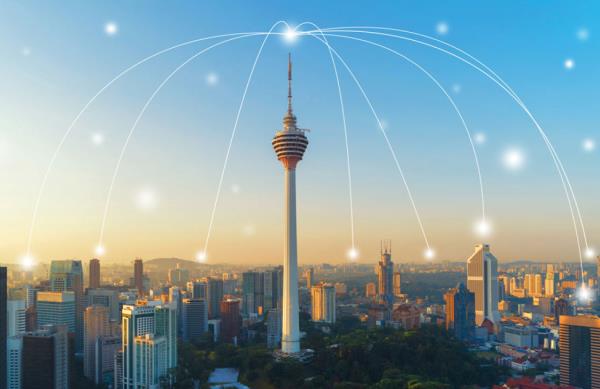
Classical and Generative AI are reshaping telecommunications as we know it – so what do operators need to know to make the most of these new capabilities?
AI has already significantly reshaped the telecommunications landscape in a very short period by helping telcos improve service quality, increase customer satisfaction and develop new products and services.
“Communications service providers (CSPs) are now showing a great deal of interest in AI and ML in general to accelerate the transformation of enterprises. AI has applications in literally hundreds of areas of a CSP’s operations, but it is frequently unclear where those applications will have the greatest impact,” notes Prianca Ravichander, chief marketing officer, Tecnotree. “The telecom industry has seen several changes as a result of the introduction of GenAI, including improved customer experiences, network management, and service delivery.”
While GenAI has gotten a lot of attention recently, “what’s interesting about the latest AI innovations is seeing how they can accelerate outcomes and open new opportunities,” shares Abhishek Sandhir, managing director, telecommunications, Sand Technologies. “For example, we’ve recently partnered with one of the world’s largest MNOs to combine classical AI applications with GenAI to take their capabilities and growth strategies to the next level. AI has been reshaping the telecom landscape for many years, but the possibilities for new AI-related advances and AI-generated business opportunities are quite exciting.”
Indeed, the opportunity to personalise the customer experience is rising to a new level with AI, and for telecom providers who have gold mines of customer behavior data and network performance data, the impact of AI is limitless. “The ability to both customise offers and automate the execution of each customer’s needs has the potential to drive new revenue and reduce costs,” reports Joy King, vice president, Optiva.
Many telcos are deploying AI-powered chatbots and virtual assistants to provide instant customer support, resolving routine issues with little to no human intervention while freeing up human agents for more complex or higher value tasks.
“What’s really exciting is how generative AI is now completely changing how CSPs can build and launch new products and services through its natural language and image recognition capabilities. This is transforming how CSPs translate new product ideas into commercial offerings, dramatically accelerating the process from concept to catalogue,” adds Dominic Smith, marketing director, Cerillion.
Holistic optimisation
AI is playing a growing role in helping MNOs in southern Asia and the world at large address the constant challenges of network congestion, competition, churn, fraud, and sustainability.
“AI accomplishes nothing without a strong data foundation, and MNOs are some of the most data-rich organisations in the world,” says Sandhir. “The sheer number of customers and the volume of network-enabled engagements they generate present operators with a huge number of optimisation and improvement possibilities. Their challenge is capturing, analysing and gaining insights from that data, which is where AI plays an essential role.”
The biggest challenge that MNOs face, especially the largest ones, is volume, asserts King, and since “network performance can be impacted by so many variables, with AI, the ability to evaluate and act on issues including fraud and cybersecurity risks becomes exponentially more achievable due to the scope, scale and speed of proactive AI evaluation and actions.”
Indeed, network optimisation is an obvious use case for AI, but MNOs must go beyond simple adjustments to look more holistically at how their networks operate.
“AI can help identify ways to adjust azimuth, angles and power levels based on real-time demand, which helps to prolong equipment life while reducing energy consumption - a cost-saving AND a sustainability story,” explains Sandhir.
“AI Predictive Analytics uses historical data to predict when network congestion is likely to occur and takes proactive measures to prevent it,” confirms Ravichander. “It anticipates traffic spikes and manages resources dynamically to ensure optimal performance. AI-Driven Resource Management also allocates bandwidth and other network resources in real-time based on current demand and predicted future needs, distributing network traffic efficiently across available resources to prevent bottlenecks. Self-optimising networks (SONs) implement AI to continuously monitor and adjust network parameters for optimal performance. Deploying AI at the edge to process data locally reduces latency and congestion in the core network.”
“Used in other ways, AI can review and analyse back-office data in a fraction of the time it takes a team of humans,” adds Sandhir. “This delivers efficiency gains, but it can also generate insights that uncover new marketing strategies to reduce churn and illuminate trends that indicate (and mitigate) fraudulent behaviour.”
Ravichander elaborates on the fraud factor, explaining that real-time monitoring with AI monitoring transactions and network activities enables the detection and prevention of fraudulent activities: “pattern recognition uses ML to identify patterns and anomalies indicative of fraud. Fraud prevention is bolstered by automated response systems that swiftly respond to detected fraud attempts, minimising impact.”
Making money
Telecom operators have long sought more ways to monetise their networks, particular amidst increasing competition and falling core service revenues. Additionally, MNOs make significant CapEx investments to build market-leading networks, and finding new and faster paths to monetise those networks is critical.
“Oftentimes MNOs already have the data they need to identify new monetisation opportunities. What they’re missing is the capability to unlock that data and gain business insights from it,” explains Sandhir. “AI can help operators quickly generate business intelligence from data and combine those insights with demographic, population and demand projections that help shed light on new business opportunities. Specific recommendations will always vary by operator and location, but even the simplest insight can inform the development and launch of services that monetise networks in new and exciting ways.”
Most telcos face significant challenges in translating a marketing idea for a new product or promotion into the corresponding BSS configuration needed for monetisation.
“In fact, many a new product never gets off the drawing board because everyone knows it will take too long to build, test and launch, stifled by legacy systems and processes,” says Smith. “GenAI is a game-changer which allows telcos to build and launch new products and services faster than ever before by breaking down the barrier between the marketing and operational teams. Telcos can go straight from whiteboard brainstorming to product testing and validation, using GenAI to translate natural language and images into the required catalogue configuration in a matter of seconds.”
“Today, when customers need new services, new plans, or new devices, they begin their own research and reach out to their current provider - or seek new options. With GenAI, identifying customers’ interests and making proactive offers represents new revenue opportunities. Much like the ads that follow us all around the internet when we search for something or hover over an image for just a few seconds, telcos can capitalise on these early interest signs and take action,” agrees King.
“CSPs can now take on the digital service providers at their own game, using GenAI to power a fail-fast approach to launching and monetising new products and services,” adds Smith.
The conversation around AI often focuses on optimisation and efficiency, and while those are legitimate ways to impact the bottom line, there’s no limit to the number of ways CSPs can use AI to generate new revenue.
“On the deployment front, AI can help operators predict with confidence the most-profitable fibre and wireless sites based on variables that can be further evaluated using a network digital twin,” says Sandhir.
“By using AI to optimise network performance, outages that completely shut down operations can be avoided. AI can predict which locations would see the highest interest usage at a given moment, using predictive and historical data. The network can prevent slowdowns and failures by boosting service to areas where it anticipates a spike in traffic,” adds Ravichander – again, increasing customer satisfaction and cutting churn.
Innovative CSPs are now pursuing a strategy that involves collaborating with ecosystem partners to provide additional offerings and expand into new and adjacent markets.
“It is predicted that new go-to-market ecosystems, driven by partnerships and a move away from vertical integration and towards open ecosystems, will accelerate growth beyond connectivity. To onboard ecosystem services, CSPs will implement open platform architectures that are data-driven and user-centric,” says Ravichander. “To increase long-term value, telcos are collaborating with companies in related industries to build next generation use cases.”
Obstacles to adoption
Amongst all the headlines, innovations and fanfare, the real-world incorporation of AI into mobile networks has been limited to date.
“The technology still seems intimidating to a number of people. As a result, one of the biggest hurdles to broader AI adoption is simply fear of the unknown,” explains Sandhir. “Some of the largest operators have deployed AI for years, but quite a few are still wondering how best to use AI. They’re not questioning whether they should use AI; they’re just not sure how to use it or where to begin. That inherently causes apprehension and delay. Finding an experienced partner that’s worked at the intersection of telecom and AI is a great first step for an MNO to adopt AI to improve their business strategy.”
King highlights that the talent needed to integrate AI into the telco network is in high demand – “and the telco industry isn’t where most of the AI experts are choosing to go. Secondly, the regulatory scrutiny that MNOs face is difficult to navigate, especially because regulators themselves are still struggling to fully understand AI. And finally, the investment needed (both in human resources and technology) is significant, and with the slowdown in revenue growth and profitability, many MNOs are reluctant to take this important next step. But without moving forward boldly, MNOs risk losing another round of opportunity to new market entrants and the hyperscalers.”
Ravichander believes that MNOs are reluctant to use AI to enhance their business strategy for several reasons, including the high cost of implementation. Many MNOs, especially smaller ones, may find it difficult to make the significant upfront investments in technology, infrastructure, and trained personnel that are frequently needed to deploy AI solutions. The complexity of integration presents an additional challenge as many MNOs have outdated systems that are difficult to integrate with current AI technologies.
“The management and quality of data pose significant challenges as well. AI is strongly dependent on high-quality data, but poor data quality, inconsistent data formats, and data silos affect many MNOs,” says Ravichander. “These problems have the potential to reduce AI applications’ effectiveness and impede their deployment.”
AI adoption is further complicated by privacy and regulatory issues, adds Ravichander: “large volumes of personal data are frequently handled during the implementation of AI, which raises questions around data privacy and compliance with laws. It can be difficult to navigate these legal restrictions, which could impede the use of AI technologies.”
Operators beware
Integrating AI into the network is no simple task – and not one that should be viewed as a short-term project.
“Integrating AI into the network is a big step for operators, and to guarantee a successful deployment, they must first clearly define their goals and use cases such as network optimisation, customer service enhancements, and predictive maintenance,” says Ravichander. “Setting high-impact topics as priorities can show early success and generate momentum. Yet another crucial component is data management. Poor data quality might result in ineffective AI models, it is imperative to ensure that data is clean, accurate, and consistent.”
According to Sandhir, operators must keep in mind that any AI application must be built on a solid data foundation to be successful; if the data sources are low quality, the AI recommendations will be too. Secondly, successful AI implementation is as much about the human element as it is about the technology. Especially with AI, where people are concerned about job displacement, the operational and organisational mindset need to be mature enough to support the integration of AI in and around the network environment.
“Third, operators need to remember that AI is intended to complement, not replace, human ingenuity,” emphasises Sandhir. “Classical AI and GenAI can present data in new ways and uncover new insights, but it’s up to an individual or team to make the strategic decision. AI can empower MNOs to innovate, but it can’t innovate on its own.”
Understanding the benefits and real-world applications of AI networking in an organisation is beneficial, but it’s also important to grasp the options for implementation.
“Successful AI networking deployment requires a seamless integration of hardware, software, and networking technologies. To achieve the desired results of AI networking, all these components need to operate together,” says Ravichander. “It is crucial to invest in scalable and adaptable infrastructure that can meet the processing demands of artificial intelligence applications. Another crucial step is to guarantee that AI solutions can be smoothly integrated with legacy systems and current network infrastructure.”
“One of the biggest opportunities that operators have is to use their own internal data goldmines from both BSS and OSS platforms but also include external market data. Choosing to implement AI using only their own internal data is a step forward, but it’s not enough,” warns King. “Operators must ensure that AI can be trained on both internal and external data sources to maximise the impact.”